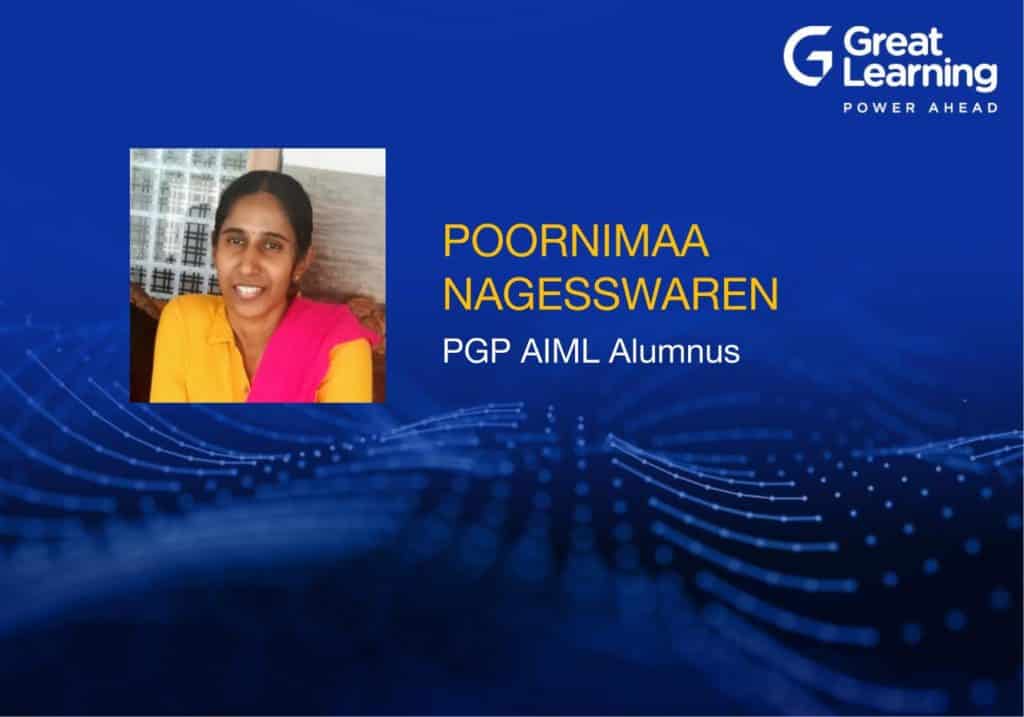
With the help of Artificial Intelligence, we can improve processes by 90% or more. Thus, taking up an AI course and upskilling can prove to be extremely beneficial for your career. Read further to learn about Poornimaa’s journey with Great Learning’s PGP Artificial Intelligence and Machine Learning Course in her own words.
I am Poornimaa, based out of Chennai. I live with my spouse and two daughters. Elder one is in 4th grade, and younger own is a two-year-old. My hobbies are cooking traditional food, Pencil drawing, mandala drawing, Paper Craftwork, and other fine arts. I have developed a recent interest in public speaking and Securities Investment and exploring them for my personal growth. At profession, I am a passionate Learner and a Problem Solver. I started my career as an application developer, worked as a Data Engineer, and now transforming into a Data Scientist in Analytics. I have exposure working in multiple domains like a Retail, airline, Investment, and Wholesale Banking and have a total of 13.5 years of experience. I am a Big Data Engineer in one of the leading MNC banks.
I was a Data Engineer building orchestration pipelines around NLP solution that was built using Market ready AI tools. Technical duties were to load the data from various sources to Hadoop Data Lake, feed data to custom model for NLP extraction, productize micro-services in Cloud, generate metrics report on the performance of the model for Risk evaluation, NLP extraction using third-party tools/products. After completing the PGP-ML program, I switched my role to ML and am now enrolled in the PGP-AI program.
One of the in-house products receives claim documents from different clients in image format in a variety of templates. The problem is to identify and classify the templates, do OCR, extract attributes, and automate the end-to-end pipeline. The key objective is to automate the whole pipeline and improve the accuracy to greater than 90%.
The image documents that we receive from the client are jumbled up, the documents are separated as independent pages, and our current objective is to classify each page to the appropriate document class. Other challenges are, the image documents that we receive every day in real-time are from multiple clients with varied templates across/within the classes, skewed images, watermarked pages, grayscale background, overlapping fonts within the document. A major problem here is OCR accuracy, data Integrity, imbalanced classes, mislabeled classes, and Data Extraction.
Classifying a document image to a particular class is crucial for both technology and business. Model prediction accuracy at the initial stage should score a good level of confidence to progress further. Otherwise, all downstream model components in the pipeline will suffer in terms of performance. If the solution cannot be implemented in-house, then Organization has to buy in other AI-powered tools from the market, which comes with a high cost.
We used Tesseract for OCR, Image enhancement using OpenCV, LinearSVC for classification, Stratified k-fold for cross-validation. Exploratory data analysis has brought greater insights to understand the data. At the first attempt, I manually explored majorly misclassified labels and understood the rules for information classification. Then identified the misclassification is due to middle/end pages which are actually separated from its first/parent page document. The data integrity between the scanned pages within a document is lost. This should be taken care of during Data pre-processing technique.
Firstly, the scattered images are grouped into a single document so that the document title of orphan pages is tagged to its parent. In this way, all Orphan documents are classified correctly and lifted the accuracy from 20% to 81%. Secondly, validate the output of the model to capture the errors. The volume of training data (50k+ records) provided for the model building was a little high, and it was tough to catch up and categorize errors. Stratified k-fold Cross-validation is used to drill down the errors from the entirety. Took test data from each fold narrowed down misclassified classes. One of the classes was incorrectly annotated, and the remaining incorrect classes are due to noise. We fixed the annotation and removed the noise during Pre-processing. The accuracy lifted to 90%.
A change in the accuracy has impacted the progress of our POC to the next step, Data Identification/Extraction. Efforts are made to provide an AIML solution rather than purchasing a vendor-based tool for Data Identification and Capturing. The benefits are cost-saving to the Organization and create more opportunities in AIML space for the division.
I just started a beginner step in ML, waiting to learn and practice AI (Image processing, NLP, and Deep Learning) to address the above solution. Overall, this exercise helped me to document and summarize Learning/problem/solution and an approach to market myself.