- Great Learning
- Free Courses
- Machine Learning
Bias Variance Tradeoff
Ratings
Level
Learning hours
Learners
Earn a certificate of completion
Get free course content
Learn at your own pace
Master in-demand skills & tools
Test your skills with quizzes
Skills you will learn
About this course
In simple words, bias means how far you have come in predicting the desired value from your actual value. It is an approach that can ultimately make or break the model in favor or against your idea. It can also be thought of as a methodological error in your machine learning model due to inappropriate assumptions made by you during the making of that model. Variance is the reverse of bias, It is called the variance when your model performs exceptionally well on the training dataset yet fails to live up to the same standards when running it on an entirely new dataset. The trade-off is simply the tension that exists between the error that is introduced by bias and variance.
Why upskill with us?
Course Outline
Earn a certificate of completion
Get free course content
Learn at your own pace
Master in-demand skills & tools
Test your skills with quizzes
Learner reviews of the free Machine Learning course
5.0
5.0
What our learners enjoyed the most
Skill & tools
62% of learners found all the desired skills & tools
Frequently Asked Questions
Will I receive a certificate upon completing this free course?
Is this course free?
Will I get a certificate after completing this Bias Variance Tradeoff free course?
Yes, you will get a certificate of completion for Bias Variance Tradeoff after completing all the modules and cracking the assessment. The assessment tests your knowledge of the subject and badges your skills.
How much does this Bias Variance Tradeoff course cost?
It is an entirely free course from Great Learning Academy. Anyone interested in learning the basics of Bias Variance Tradeoff can get started with this course.
Is there any limit on how many times I can take this free course?
Once you enroll in the Bias Variance Tradeoff course, you have lifetime access to it. So, you can log in anytime and learn it for free online.
Can I sign up for multiple courses from Great Learning Academy at the same time?
Yes, you can enroll in as many courses as you want from Great Learning Academy. There is no limit to the number of courses you can enroll in at once, but since the courses offered by Great Learning Academy are free, we suggest you learn one by one to get the best out of the subject.
Why choose Great Learning Academy for this free Bias Variance Tradeoff course?
Great Learning Academy provides this Bias Variance Tradeoff course for free online. The course is self-paced and helps you understand various topics that fall under the subject with solved problems and demonstrated examples. The course is carefully designed, keeping in mind to cater to both beginners and professionals, and is delivered by subject experts. Great Learning is a global ed-tech platform dedicated to developing competent professionals. Great Learning Academy is an initiative by Great Learning that offers in-demand free online courses to help people advance in their jobs. More than 5 million learners from 140 countries have benefited from Great Learning Academy's free online courses with certificates. It is a one-stop place for all of a learner's goals.
What are the steps to enroll in this Bias Variance Tradeoff course?
Enrolling in any of the Great Learning Academy’s courses is just one step process. Sign-up for the course, you are interested in learning through your E-mail ID and start learning them for free online.
Will I have lifetime access to this free Bias Variance Tradeoff course?
Yes, once you enroll in the course, you will have lifetime access, where you can log in and learn whenever you want to.
Become a Skilled Professional with Pro Courses
Gain work-ready skills with guided projects, top faculty and AI tools, all at an affordable price.

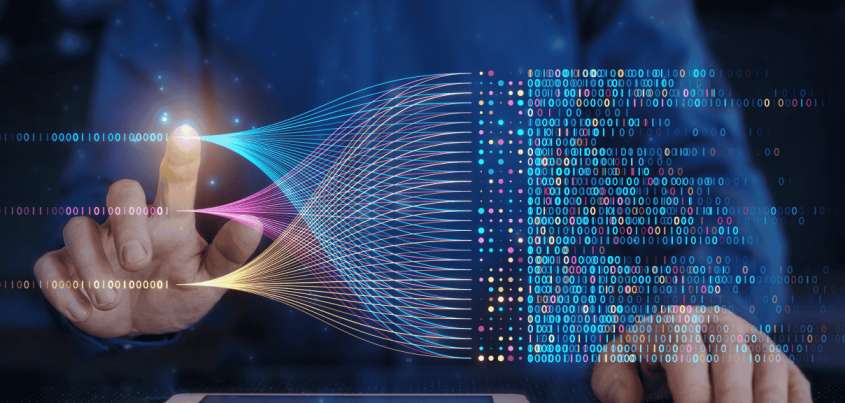
View Course

Included with Pro Subscription

.png)
View Course

Included with Pro Subscription

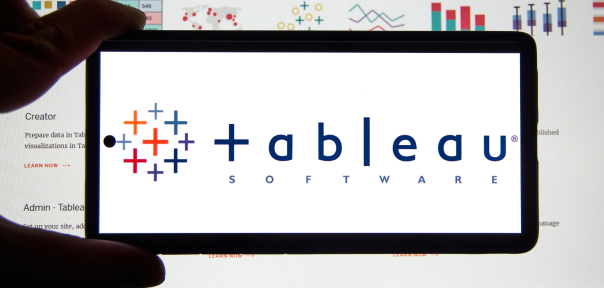
View Course

Included with Pro Subscription

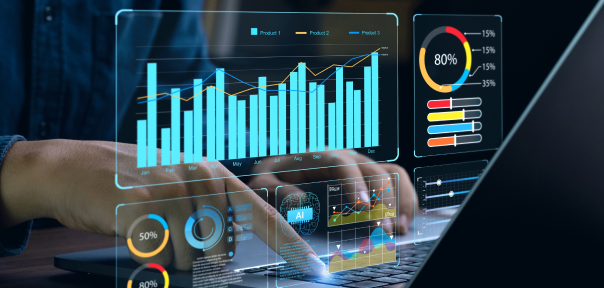
View Course

Included with Pro Subscription

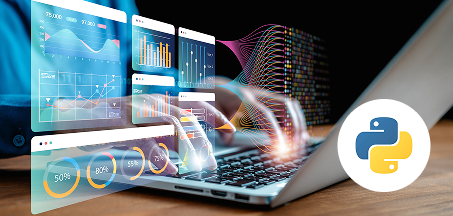
View Course

Included with Pro Subscription

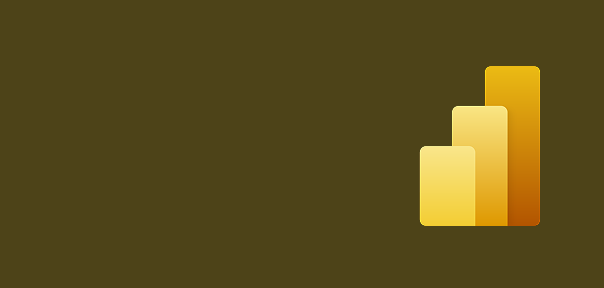
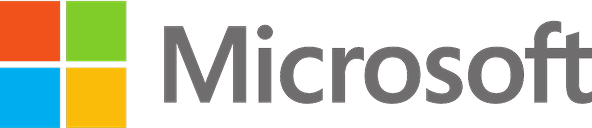
View Course

Included with Pro Subscription

.png)
View Course

Included with Pro Subscription

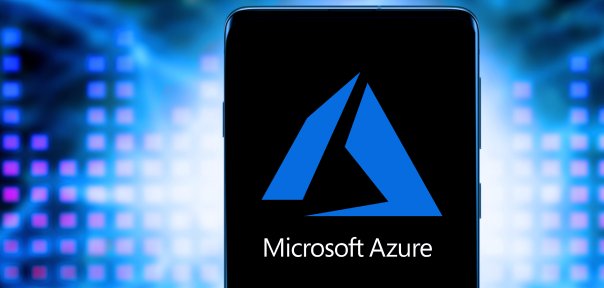
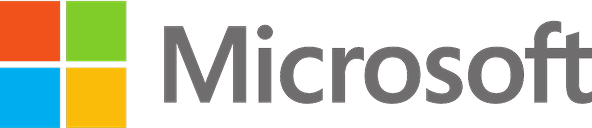

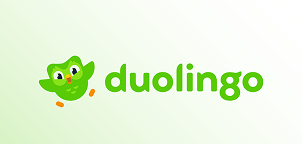
View Course

Included with Pro Subscription

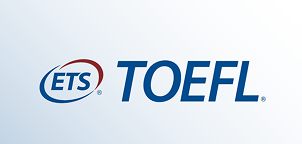

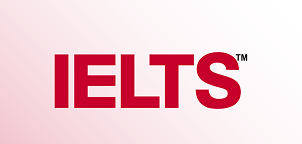

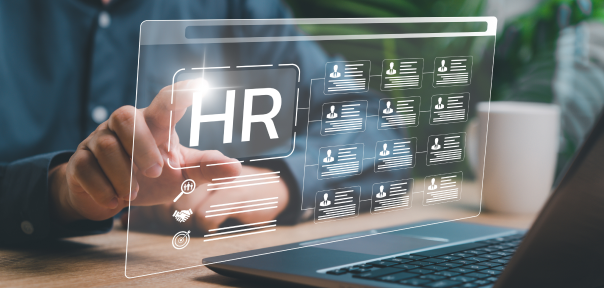
View Course

Included with Pro Subscription

.png)
View Course

Included with Pro Subscription

.png)
View Course

Included with Pro Subscription

.png)

 (1).jpg)
View Course

Included with Pro Subscription

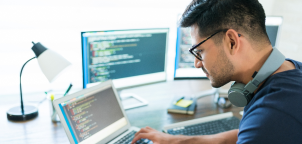
View Course

Included with Pro Subscription

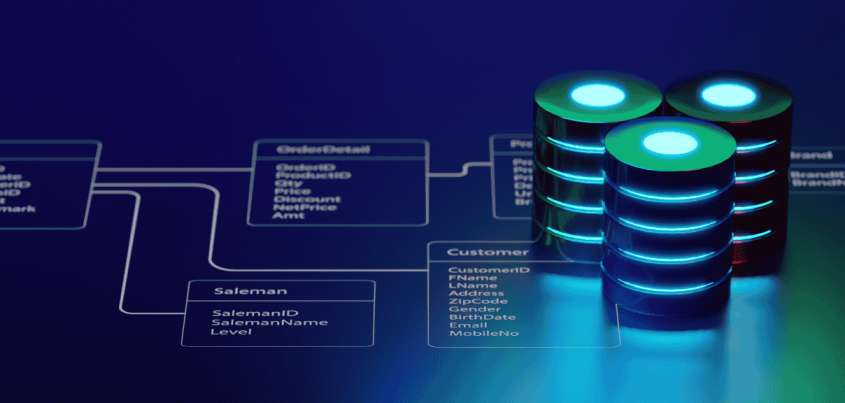
View Course

Included with Pro Subscription

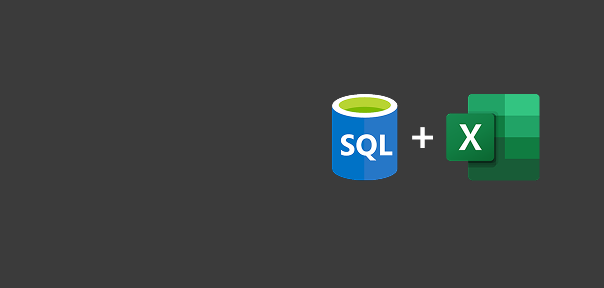
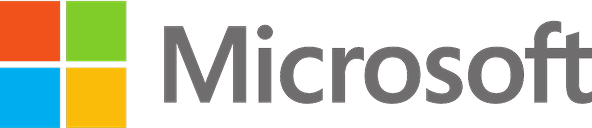
View Course

Included with Pro Subscription

 (1).png)
View Course

Included with Pro Subscription

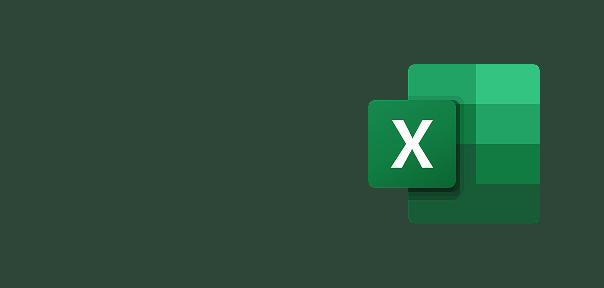
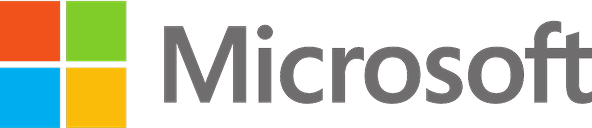
View Course

Included with Pro Subscription

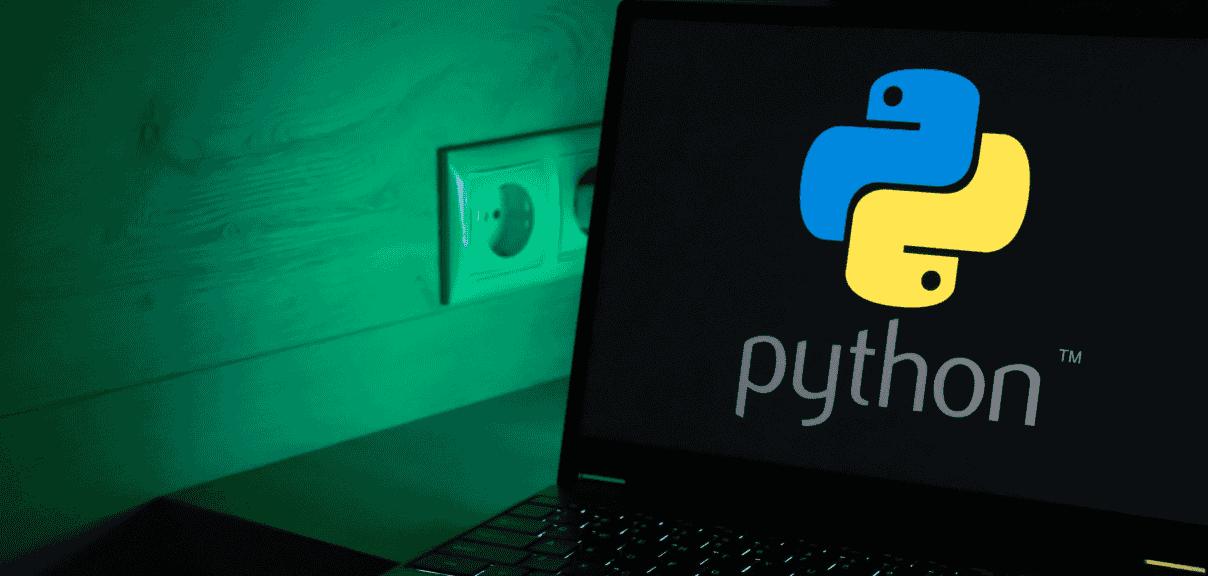
View Course

Included with Pro Subscription

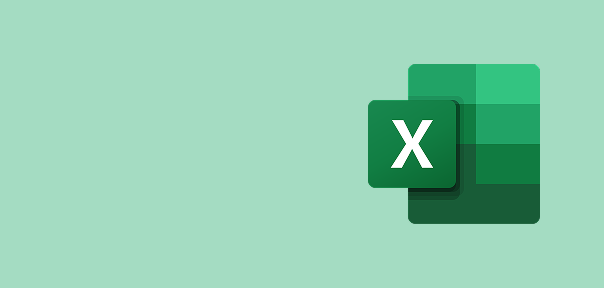
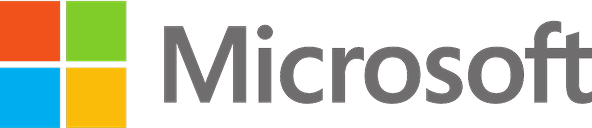
View Course

Included with Pro Subscription

.png)
View Course

Included with Pro Subscription

.jpg)
View Course

Included with Pro Subscription

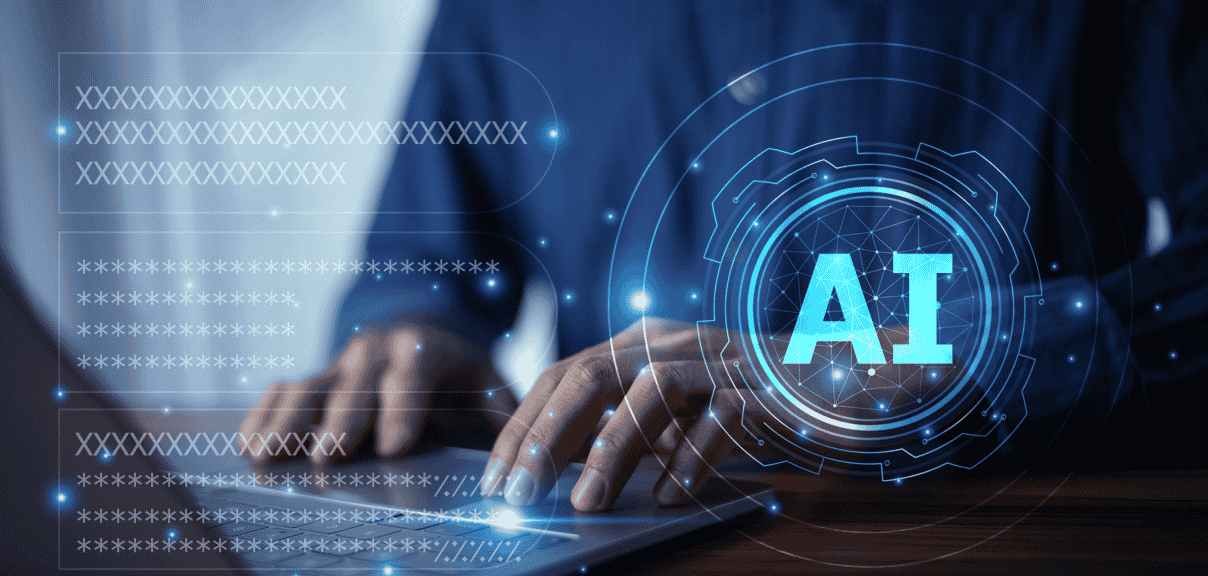
View Course

Included with Pro Subscription

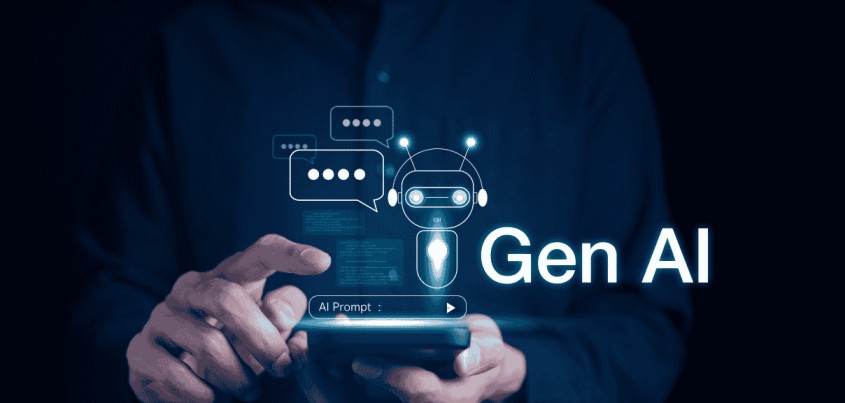
View Course

Included with Pro Subscription
-
Data Science & ML
PRO
136 coding exercises 6 projects17 hrs video contentView Course
Included with Pro Subscription
PRO
1 coding exercise 1 project12 hrs video contentView Course
Included with Pro Subscription
PRO
1 project8.5 hrs video contentView Course
Included with Pro Subscription
PRO
40 coding exercises 3 projects3.5 hrs video contentView Course
Included with Pro Subscription
PRO
1 coding exercise 1 project12.5 hrs video contentView Course
Included with Pro Subscription
PRO
1 project7.5 hrs video contentView Course
Included with Pro Subscription
-
Cloud Computing
PRO
1 project8.58 hrs video contentView Course
Included with Pro Subscription
PRO
-
Test Preparation
PRO
7.5 hrs video contentView Course
Included with Pro Subscription
PRO
PRO
-
Management
PRO
1 project10 hrs video contentView Course
Included with Pro Subscription
PRO
1 project7.5 hrs video contentView Course
Included with Pro Subscription
PRO
2 projects9 hrs video contentView Course
Included with Pro Subscription
PRO
PRO
2 projects9.5 hrs video contentView Course
Included with Pro Subscription
-
IT & Software
PRO
1 project10 hrs video contentView Course
Included with Pro Subscription
PRO
39 coding exercises 2 projects5 hrs video contentView Course
Included with Pro Subscription
PRO
39 coding exercises 4 projects8.5 hrs video contentView Course
Included with Pro Subscription
PRO
3 projects16.05 hrs video contentView Course
Included with Pro Subscription
PRO
2 projects10 hrs video contentView Course
Included with Pro Subscription
PRO
51 coding exercises 3 projects11.5 hrs video contentView Course
Included with Pro Subscription
PRO
2 projects5.5 hrs video contentView Course
Included with Pro Subscription
-
Cyber Security
PRO
1 project7.5 hrs video contentView Course
Included with Pro Subscription
-
AI & Generative AI
PRO
2 projects12 hrs video contentView Course
Included with Pro Subscription
PRO
18 coding exercises 3 projects12.5 hrs video contentView Course
Included with Pro Subscription
PRO
10 coding exercises 3 projects9 hrs video contentView Course
Included with Pro Subscription
Subscribe to Academy Pro & get exclusive features
$25/month
No credit card required
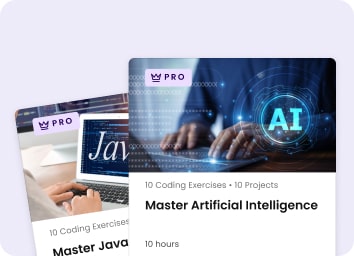
20+ Pro courses
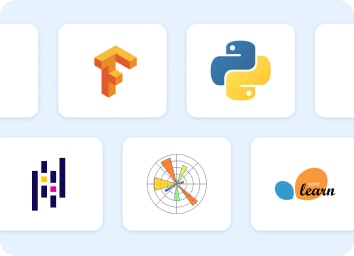
200+ coding exercises with AI support
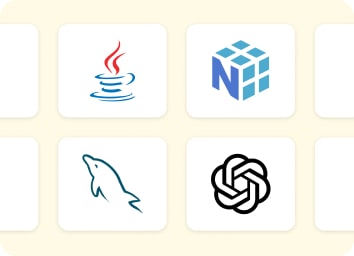
30+ hands-on guided projects
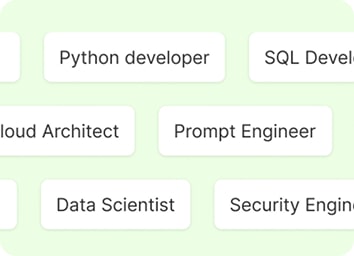
AI mock interviews
Recommended Free Machine Learning courses

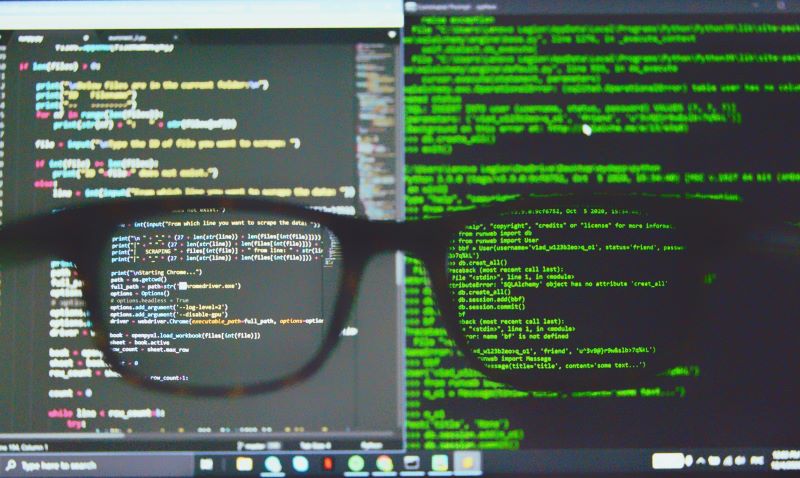

.jpg)
Similar courses you might like

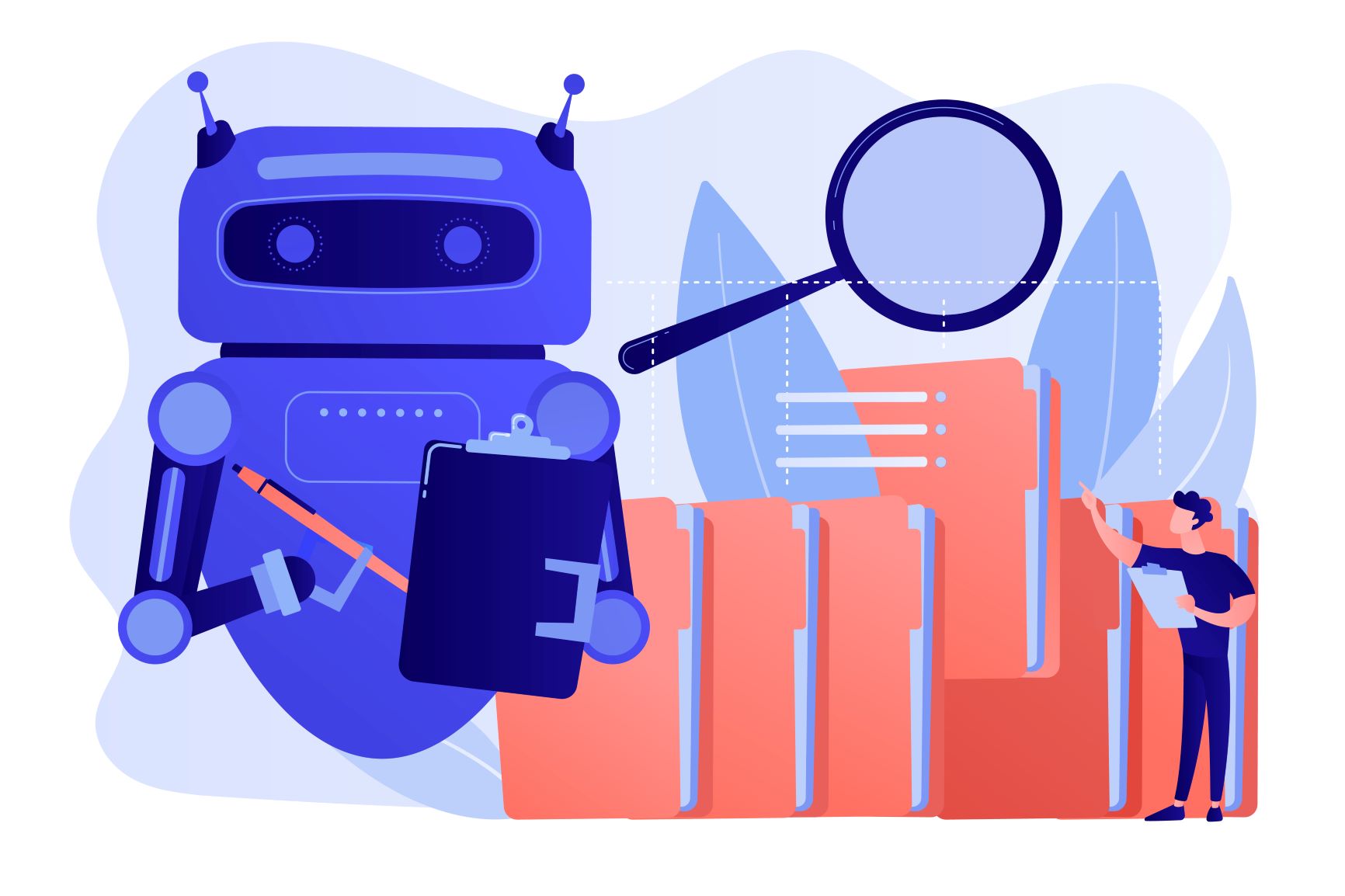

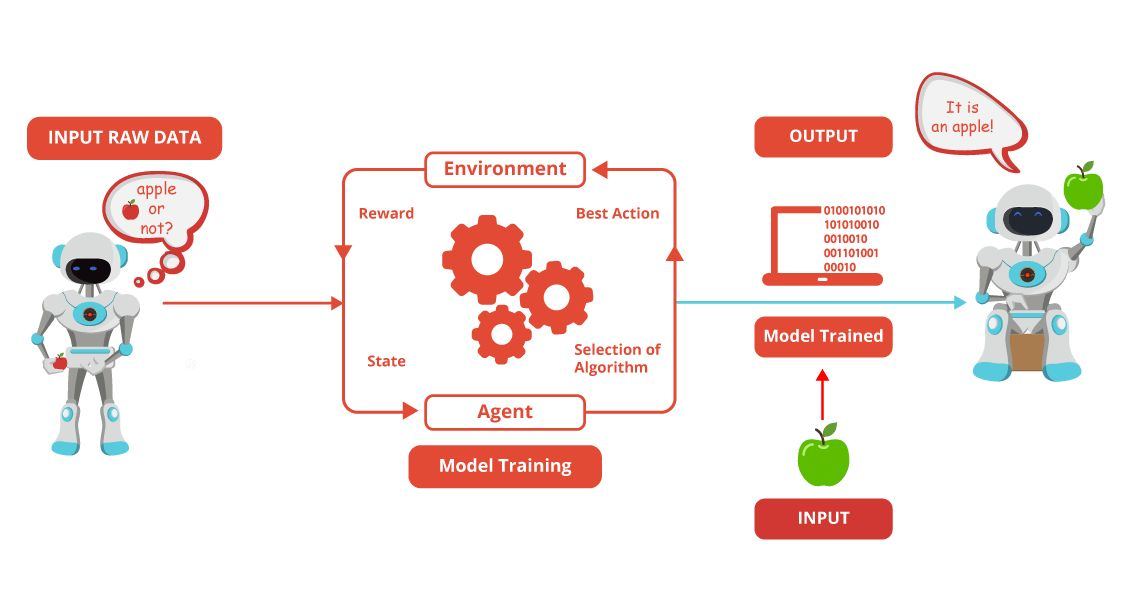

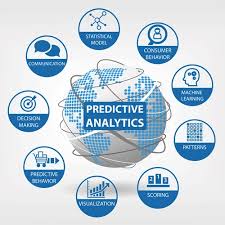

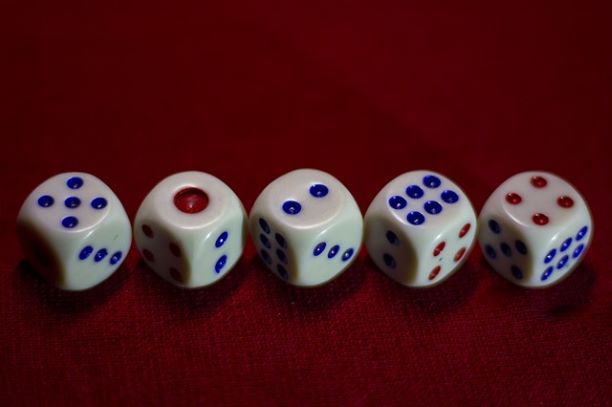
View Course
Related Machine Learning Courses
-
Personalized Recommendations
Placement assistance
Personalized mentorship
Detailed curriculum
Learn from world-class faculties
50% Average salary hike -
Johns Hopkins University
Certificate Program in AI Business Strategy10 weeks · Online
Know More
-
Walsh College
MS in Artificial Intelligence & Machine Learning2 Years · Online
Know More
-
MIT Professional Education
No Code AI and Machine Learning: Building Data Science Solutions12 Weeks · Online · Weekend
Learn from MIT FacultyKnow More